Abstract
Federated learning (FL) has been a promising approach in the field of medical imaging in recent years. A critical problem in FL, specifically in medical scenarios is to have a more accurate shared model which is robust to noisy and out-of distribution clients. In this work, we tackle the problem of statistical heterogeneity in data for FL which is highly plausible in medical data where for example the data comes from different sites with different scanner settings. We propose IDA (Inverse Distance Aggregation), a novel adaptive weighting approach for clients based on meta-information which handles imbalanced and non-iid data. We extensively analyze and evaluate our method against the well-known FL approach, Federated Averaging as a baseline.
Our Contributions:
- A novel aggregation method for federated learning
- Robustness to non-IID data
- Robustness to overfitting or poisonous clients
Link to the paper: SpringerLink | arXiv
Link to the code on github: Code (will be published soon)
Team
Our team at TUM
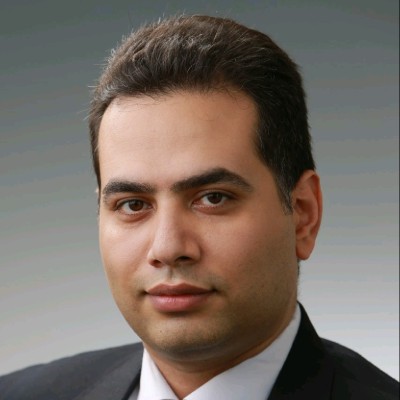
Yousef Yeganeh
Research Scientist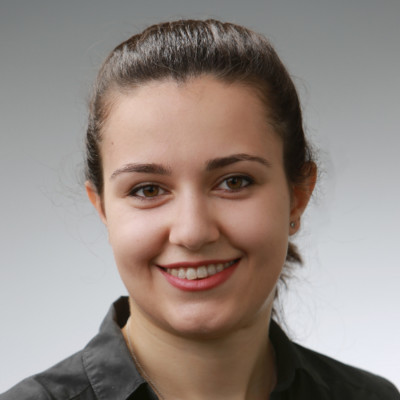
Azade Farshad
Research Scientist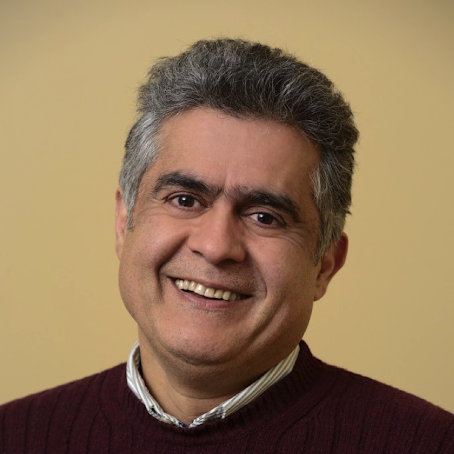
Prof. Dr. Nassir Navab
Professor, Team Lead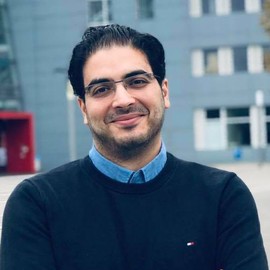